Natural Language Processing (NLP) stands at the forefront of AI-powered technology, revolutionizing our approach to both text and audio data. Consider a computer analyzing a book and identifying its themes. Or a smart system evaluating customer feedback from numerous reviews. The advent of NLP models like BERT and GPT marks a significant leap. They enable machines to process and understand human language with remarkable efficiency.
These models serve as foundational elements in deciphering vast quantities of text data. Their applications span various sectors, from enhancing customer service to advancing academic research. In this article, we’ll explore the capabilities of these NLP models and what makes them so powerful.
Understanding NLP Models and How They Work
Recent statistics show that deploying NLP-enabled predetermined tasks may lower customer support expenses by up to 30%. This impressive efficiency stems from the evolution of natural language processing models. They have transitioned from simple rule-based algorithms to complex, learning-based systems. These advanced models can understand, interpret, and respond to human language in a way that was once unimaginable.
Understanding these NLP models begins with recognizing their two core components:
- Data input. The model receives text data, which can range from social media posts to lengthy documents.
- Processing and analysis. The model applies algorithms to analyze this data, identifying patterns, meanings, and sentiments.
Central to refining these models is data annotation, a process where text data is meticulously labeled to train the model. This involves marking specific language elements like tone, intent, and semantic fields. By leveraging high-quality natural language processing services, these models gain an ability to comprehend human language.
A few key applications of NLP models in text analysis include:
- Sentiment analysis. Determining the emotional tone behind a series of words.
- Text classification. Categorizing text into predefined groups based on its content.
- Language translation. Converting text from one language to another accurately.
As these models have advanced, their ability to extract meaningful information has grown exponentially. They no longer just read text. They understand context, sarcasm, and even cultural references. This evolution marks a significant leap in how we process vast amounts of data. By leveraging these sophisticated NLP models, businesses can unlock insights from text data. And now they can do it faster and more accurately than ever before.
Comparative Analysis of Top 3 NLP Models
Let's delve into a comparative analysis of three prominent NLP models. These models are at the forefront of NLP, offering distinct capabilities in text data processing and analysis.
- BERT (Bidirectional Encoder Representations from Transformers) has revolutionized NLP. It processes text from both directions, unlike older models that read linearly. This dual approach helps BERT understand context and nuances in language. It excels in tasks needing a strong grasp of language semantics, like interpreting word meanings based on context.
- GPT (Generative Pretrained Transformers) is a powerhouse in text generation. Its design allows it to predict the next word in a sentence, enabling it to produce text that closely resembles human writing. This capability makes GPT a valuable tool for a wide range of applications. Its strength lies in generating coherent and contextually appropriate text. This is a significant advancement over earlier models that often struggled with these aspects.
- Transformer-based Architectures are known for their efficiency in processing large volumes of text. These models use 'self-attention' mechanisms, allowing them to process each word in relation to the entire sentence. As well as understand long-range dependencies in text. Transformer architectures are used in tasks that involve language translation and document summarization. In these cases, understanding the broader context and nuances of language is crucial.
As NLP evolves, models like BERT, GPT, and Transformer-based architectures are significant milestones. They show how progress in NLP technologies can improve our ability to get insights from text. It's important to understand their strengths and how they're used. This knowledge is key for anyone wanting to use NLP in their work or research. Such models offer nuanced ways to analyze and understand textual data, marking a leap in our ability to handle human language.
NLP Models in Action: Real-World Applications and Future Trends
NLP's practical applications span a diverse range of industries. Each aspect showcases unique ways this technology is making a tangible impact. Let's explore where it’s applicable:
- Customer service. Imagine chatbots that get both the words and emotions in customer queries. NLP-driven chatbots are changing customer support with personalized, quick responses. For example, using a GPT-based chatbot can cut down the time it takes to solve customer complaints.
- Healthcare. In healthcare, NLP helps with early disease detection by looking at patient records. It works through unstructured text, helping doctors find risk factors for chronic diseases sooner. This leads to improved patient outcomes. By using NLP in this way, healthcare providers can spot health issues early and offer better care.
- Finance. NLP now plays a key role in detecting financial fraud, beyond just sentiment analysis. It examines the language in transaction narratives to spot fraud patterns. This helps make financial transactions more secure. By using NLP this way, financial institutions can better protect against fraud.
Another advancement is in multilingual NLP models. These models aim to understand and interpret language nuances across different languages. Such advancement is key to building global communication solutions that transcend language barriers.
Summing Up
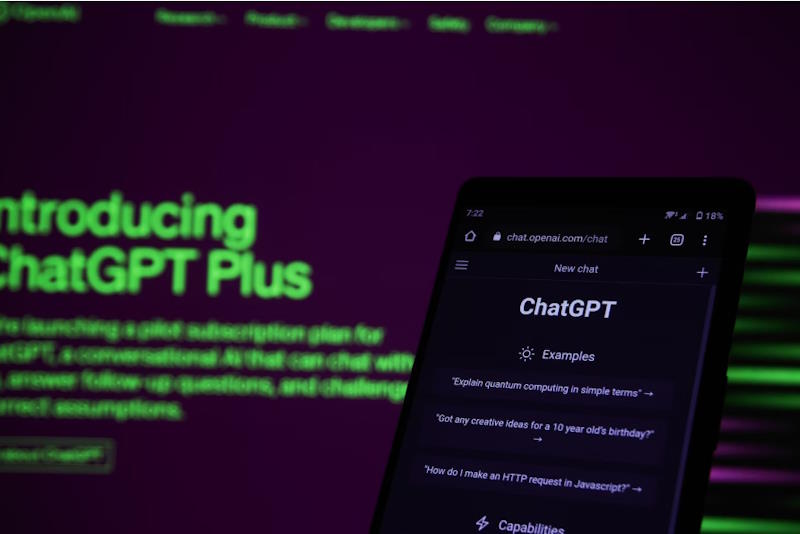
As we wrap up our exploration of NLP models, it's clear that they are gateways to understanding and leveraging the power of language in digital form. From enhancing customer experiences to making strides in healthcare and finance. But remember, the key to effective NLP lies in high-quality data annotation.
As NLP evolves, it moves towards more intuitive, human-like interactions with technology. Its uses are getting more complex to meet unique challenges in different areas. The future of NLP is about making stronger, more meaningful links between tech and human language. This shift is making technology more relatable and effective in responding to our linguistic needs.